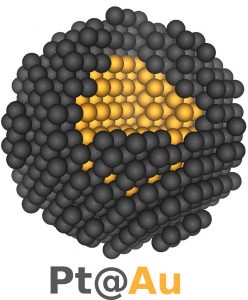
Platinum nanoparticle catalyst with a “heart” of gold
Platinum is the common catalyst material for the oxygen reduction in fuel cells and its main cost factor. With a machine learning technique, scientists from e-conversion now can forecast and optimize the performance of new catalysts with a significantly reduced amount of platinum.
Fuel cells generate electric power by converting hydrogen and oxygen into water, which renders them promising devices for sustainable energy solutions. Today, only platinum-based catalysts have appropriate activity and stability in fuel cells to drive the sluggish oxygen reduction reaction (ORR). But platinum is rare and expensive. Thus, designing catalysts with a reduced amount of platinum, is one key factor toward widespread commercialization of fuel cells. Now researchers from e-conversion have developed a novel machine learning technique to optimize the activity per mass of platinum in the oxygen reduction reaction (to publication). The team is led by Professor Alessio Gagliardi, Simulation of Nanosystems for Energy Conversion, and Professor Aliaksandr Bandarenka, Physics of Energy Conversion and Storage, both from the Technical University of Munich (TUM).
Forecasting the catalytic activity
The common catalysts are nanoparticles, which are few nanometers in size. But the chemical reaction takes place only on the surface. The general idea to reduce the platinum amount is easy to understand: a so-called core-shell structure with a thin layer of platinum on the surface and a cheaper metal inside. Various core-shell nanoparticles have already been experimentally produced already. However, the details matter. For example, the nanoparticle size, the type of core metal and the platinum layer thickness influence the distances between atoms in the entire nanoparticle. In other words: Each combination induces various deformations in the atomic lattice, which can increase or decrease the activity of the oxygen reduction. Therefore, the researchers have built a machine learning approach to predict these deformations. Moreover, with their established computer model, they can now even forecast the activity of the core-shell catalysts.
A heart made of nickel
The machine learning model was trained and tested with nanoparticles, in which nickel, copper, palladium, silver and gold as core metals are combined with platinum shells with a layer thickness of about 2-3 or 3-4 atoms. The result is impressive: The computer model identifies nanoparticles with a predicted activity four times higher than currently available commercial catalysts. In detail, it turned out that nanoparticles with copper or nickel in the core show optimal activity with a diameter size of 2 nm. For silver or gold cores, a size of 3 nm is ideal. Although gold and silver are not exactly cheap either, the worldwide gold and silver resources are much larger than the platinum occurrence.
Great teamwork of theoretical and experimental scientists
“In our team we could rely on a broad expertise in catalyst modeling, synthesis and characterization”, says Marlon Rück, the main contributing author of the study. “In this way we have succeeded in building catalyst models by combining theory with experimental data to translate catalyst requirements into synthesis guidelines”. Therefore, the results pave the way for faster core–shell nanocatalyst screenings and thus a highly efficient electrocatalytic energy conversion.
(Author: Birgit Ziller)
Publication:
Oxygen Reduction Activities of Strained Platinum Core–Shell Electrocatalysts Predicted by Machine Learning. Marlon Rück, Batyr Garlyyev, Felix Mayr, Aliaksandr S. Bandarenka, and Alessio Gagliardi. The Journal of Physical Chemistry Letters 2020 11 (5), 1773-1780. DOI: 10.1021/acs.jpclett.0c00214
Contact:
Marlon Rück
Simulation of Nanosystems for Energy Conversion (Prof. Gagliardi)
Department of Electrical and Computer Engineering
Technische Universität München